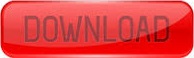
Waterfall plot: exp %>% waterfall_plot(row_idx=10)įorce (JS) plot: exp %>% force_plot(class_id = 0)ĭefine path to folders: path = 'oxford-iiit-pet' Currently summary_plot, dependence_plot, waterfall_plot, force_plot, and decision_plot are supported.įirst, get explanation object: exp = ShapInterpretation(model,n_samples = 20) Get predictions on new data: > model %>% predict(df)įastinference by Zachary Mueller has ShapInterpretation function that allows to utilize various methods within the SHAP interpretation library. Interp %>% plot_confusion_matrix(dpi = 90,figsize = c(6,6)) Plot it: interp = ClassificationInterpretation_from_learner(model) Get confusion matrix: model %>% get_confusion_matrix() =50k Implementation of OpenAI paper “An Empirical Model of Large-Batch Training” for Fastai was done by hal-314: bss = model %>% bs_find(lr=1e-3) Plot loss history: model %>% plot_loss(dpi = 200)Īt the same time, users can find optimal batch size.
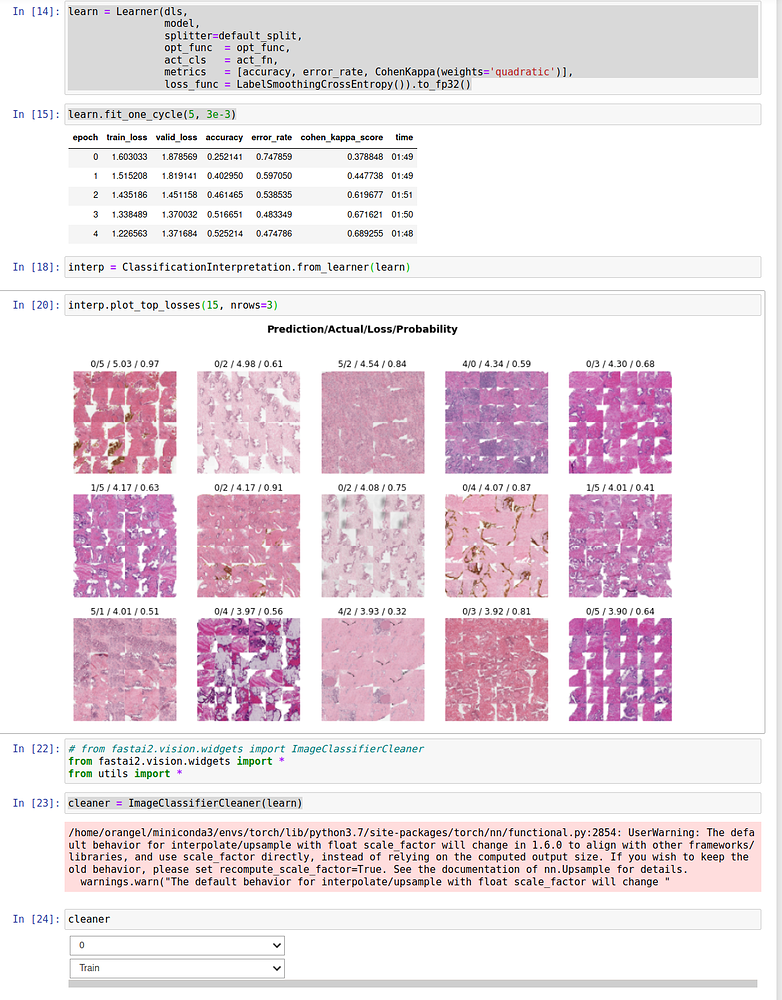
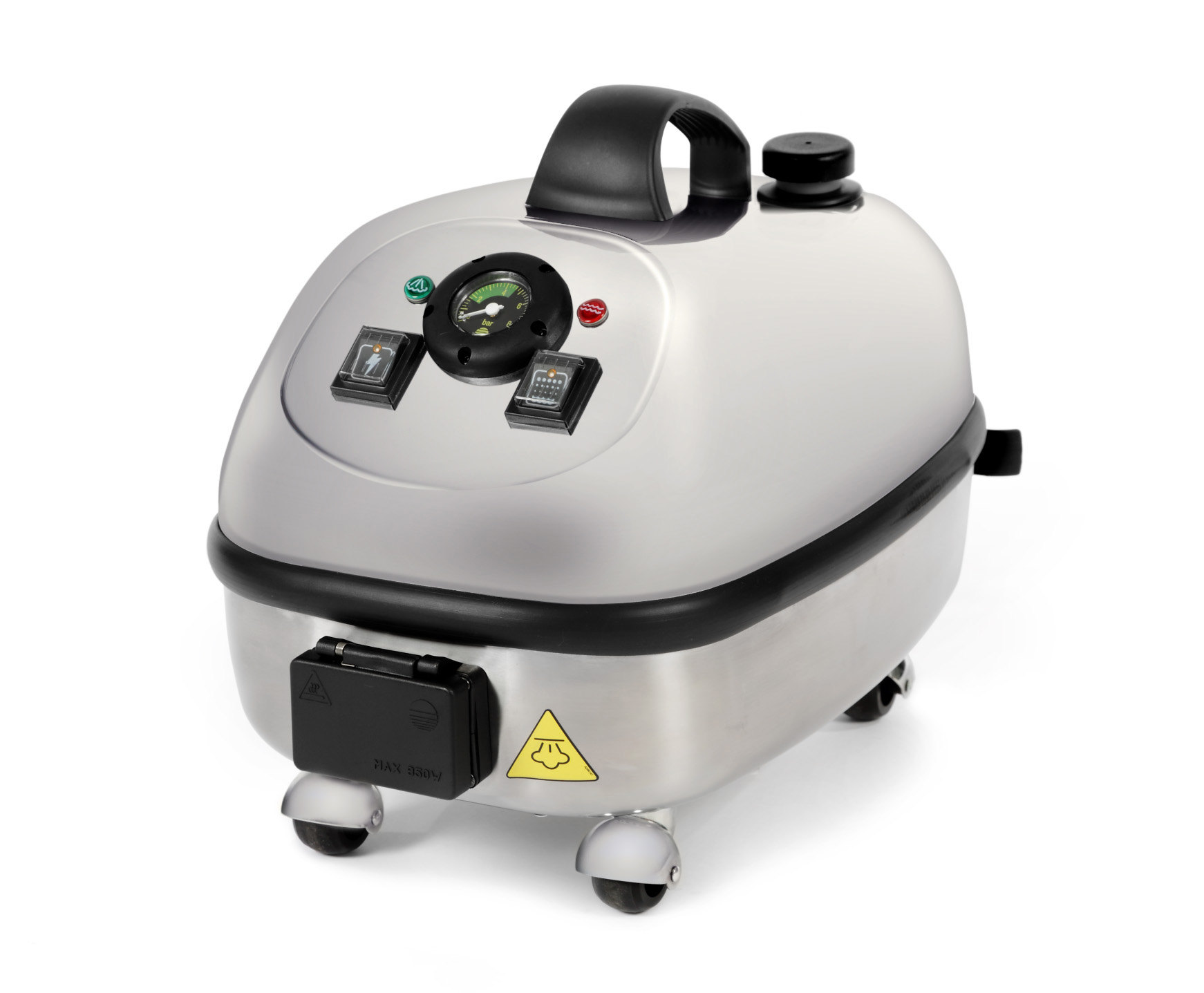
Run: model %>% fit(5, lr = 10^-1) epoch train_loss valid_loss accuracy time Loss function: FlattenedLoss of CrossEntropyLoss()īefore fitting try to find optimal learning rate: model %>% lr_find() Layer (type) Output Shape Param # Trainable Model %>% summary() TabularModel (Input shape: ) Summary: model = dls %>% tabular_learner(layers=c(200,100), metrics=accuracy) Prepare: dls = TabularDataTable(df, procs, cat_names, cont_names,
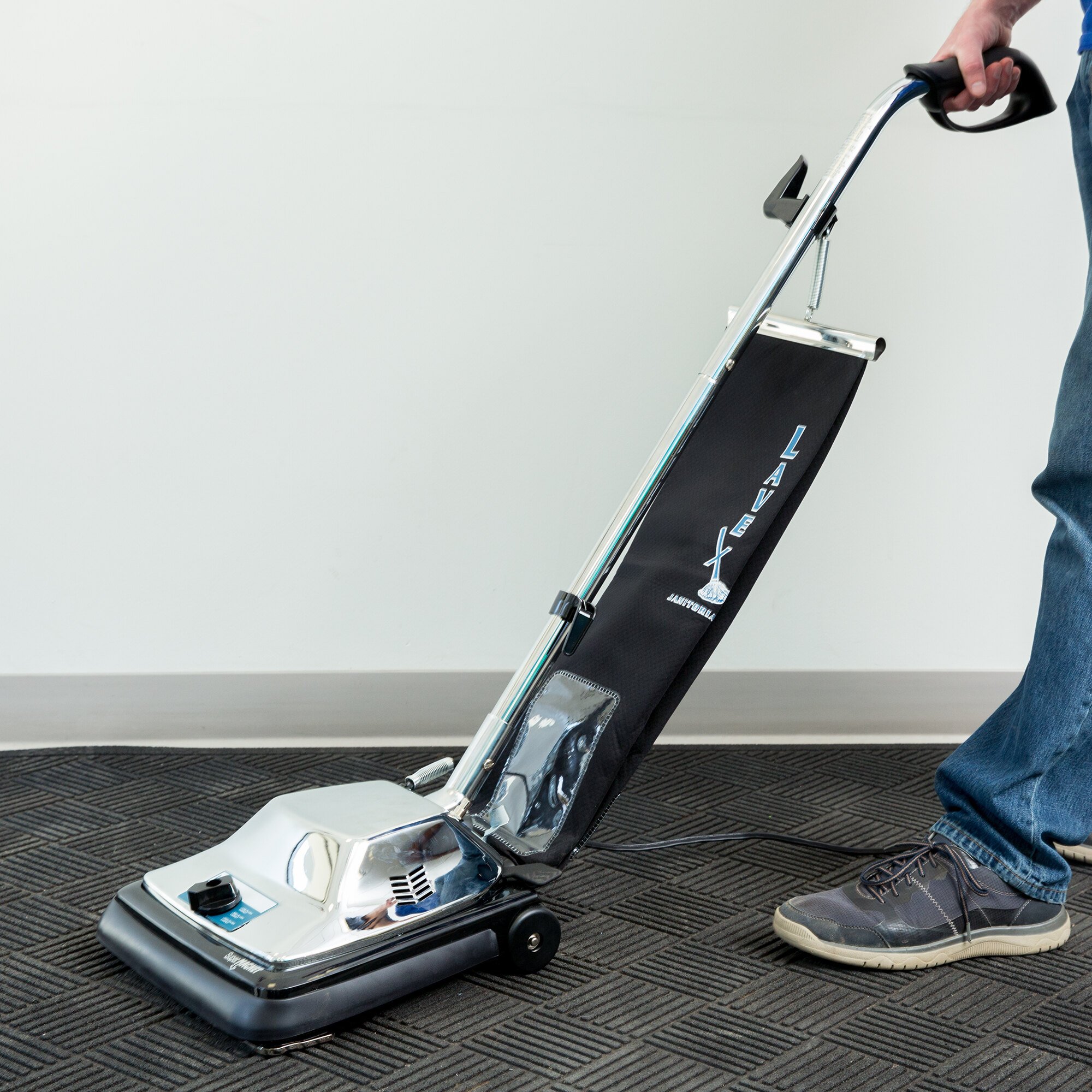
Preprocess strategy: procs = list(FillMissing(),Categorify(),Normalize()) Df = data.table::fread('adult_sample/adult.csv')Ĭat_names = c('workclass', 'education', 'marital-status', 'occupation', 'relationship', 'race')Ĭont_names = c('age', 'fnlwgt', 'education-num')
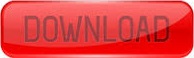